Understanding the Role of Filters in Sisense
In the realm of data analysis and visualization, the power of filters cannot be overstated. Filters allow users to refine their data, focus on specific subsets, and extract valuable insights. Sisense, as a leading business intelligence platform, offers a robust set of filtering capabilities that empower users to tailor their analyses to their precise needs. In this guide, we will delve into the world of Sisense filters, exploring their functionality, customization options, and best practices. Additionally, we'll highlight how Paldi filter plugins can enhance the filtering experience within Sisense.
Understanding Star Schema and Dimensional Modeling
Filters play a pivotal role in Sisense dashboards, serving as indispensable tools for refining and analyzing data with precision and efficiency. By allowing users to focus on specific subsets of data, filters empower them to extract actionable insights and make informed decisions. Within the context of Sisense's architecture, the importance of filters is amplified, especially in conjunction with the star schema data model.
In the star schema, dimensions are segregated into their own tables, facilitating optimized data management and query processing. This structural approach streamlines data retrieval and analysis by separating descriptive attributes (dimensions) from measurable quantities (facts), thus enhancing the clarity and efficiency of data modeling. When filters are applied within Sisense dashboards, the system dynamically establishes joins between the fact table and the relevant dimension tables. This process enriches the data with contextual information, enabling users to delve deeper into their analyses while maintaining data integrity.
Behind the scenes, Sisense leverages these joins to incorporate a 'where' clause in the SQL queries generated for each widget, ensuring that the data displayed is filtered based on the user's selections. This seamless integration of filters into the querying process not only enhances the relevance and accuracy of insights presented but also optimizes query execution times, bolstering overall dashboard performance.
Furthermore, in scenarios where multiple fact tables are present, the star schema proves invaluable. Both fact tables connect to dimension tables, ensuring that when a filter is applied to a dimension, data from all relevant fact tables is filtered accordingly. This capability enables users to conduct comprehensive analyses across disparate data sources, fostering a holistic understanding of their data landscape.
Safeguarding Data Privacy in Sisense Dashboards
In the dynamic landscape of data security within business intelligence, a critical consideration revolves around presenting aggregated values to viewers while safeguarding granular data from unauthorized access. This balancing act is particularly crucial in scenarios where sensitive information, such as individual employee salaries, must be protected due to privacy and security regulations. For instance, while it's acceptable to display aggregated salary metrics like average departmental salaries or total monthly payroll, revealing individual salary details poses a significant risk. However, the introduction of filters within dashboards can potentially breach this security by allowing viewers to infer granular information. Consider a scenario within the IT department, where most employees are above 30 years old except for one individual aged below 25. If a dashboard features filters based on department and age group, viewers could easily discern details about the younger employee by filtering for "department=IT" and "age group=20-30." This unintended revelation of sensitive data underscores the importance of vigilance when selecting filter fields and crafting dashboards.
To address this challenge, organizations must exercise caution when selecting filter fields and constructing dashboards within Sisense. By carefully evaluating the potential implications of filter usage and considering the sensitivity of the underlying data, organizations can mitigate the risk of unintentional data exposure and maintain compliance with regulatory standards. Also there is a powerful plugin available, called Avoid Over Filtering offered by Paldi Solutions, aimed at enhancing data security within Sisense dashboards.
Elevating Filter Styling
Consistency in filter styling holds significant importance within Sisense as it directly impacts the overall user experience and perception of the dashboard. Filters serve as essential navigational tools, allowing users to interact with and explore data sets effectively. When filters seamlessly integrate with the dashboard's design language, users can navigate through data more intuitively, leading to improved comprehension and analysis. Moreover, visually cohesive filters contribute to a polished and professional dashboard aesthetic, reinforcing the credibility of the data presented and instilling confidence in users. In scenarios such as OEM deployments, where brand consistency is paramount, aligning filter styles with the overall dashboard design becomes even more critical, as it ensures that the user experience remains consistent across various platforms and deployments.
In Sisense, achieving consistent filter styling involves leveraging the platform's built-in customization features alongside additional plugins for enhanced flexibility. Sisense provides native options to adjust filter colors, allowing users to match filter aesthetics with other dashboard elements. However, for more advanced customization and styling capabilities, users can opt for plugins such as those offered by Paldi Solutions. Paldi's filter plugins offer extensive customization options, enabling users to tailor filter aesthetics with precision.
Enhancing User Experience with Advanced Filter Features
User experience with filters is paramount, as it can greatly enhance the efficiency and depth of data analysis. Providing additional features around filters can offer users valuable tools to navigate and analyze data more effectively. Here are some of the features that can be considered while working with filters.
1. Reset Filters
Resetting filters to their default state after applying multiple selections is a valuable feature that enhances user experience in data analysis. This functionality allows users to easily revert back to the original filter settings, providing a convenient way to start anew with their analysis. Thankfully, Sisense includes a built-in option to reset filters, ensuring users can effortlessly return to the default filter configuration whenever necessary.
2. Filter Bookmark
Filter bookmarking, or the ability to save current filter selections for future use, greatly improves user productivity and efficiency in data analysis workflows. With this feature, users can quickly apply previously saved filter configurations, streamlining the analysis process and eliminating the need to manually recreate complex filter setups. Paldi's Filter Bookmark plugin is a valuable addition to Sisense, offering users an intuitive tool for saving and recalling filter settings with ease, thereby enhancing the overall user experience within the platform.
3. Easy Filter Field Discovery
Facilitating easy discovery of specific filter fields within a dashboard is essential for improving usability, especially in scenarios where numerous filters are present. Without such functionality, users may struggle to locate specific filters, leading to frustration and inefficiency. While Sisense lacks a built-in option for this feature, external plugins such as Paldi's Filter Catalog can fill this gap.
Harnessing the Power of Sisense Filters
Sisense caters to diverse data types, seamlessly accommodating various filtering requirements.
Text Field Filters
For text dimension columns, Sisense offers a diverse range of filtering options, allowing users to include or exclude specific items, filter based on text containment or starting patterns, and more, ensuring granular control over data selection. However, for those seeking heightened customization and flexibility, Paldi's Advanced Filters plugin emerges as a compelling alternative, providing extensive options to tailor the look and feel of filter widgets.

Date Field Filters
In Sisense, date dimensions empower users to filter data across various granularities, from day to year, facilitating comprehensive temporal analysis. With functionalities like date range selection and predefined presets such as "last 3 years" or "last 12 months," users can efficiently narrow down temporal data to derive meaningful insights. However, for those seeking additional customization and ease of use, Paldi's Date Range filter plugin presents a compelling alternative.

Numeric Field Filters
Numeric dimensions afford users the flexibility to filter data based on numerical criteria, including greater than, less than, or equal to specific values. This capability, coupled with Paldi plugins, empowers users to refine their analyses with precision.
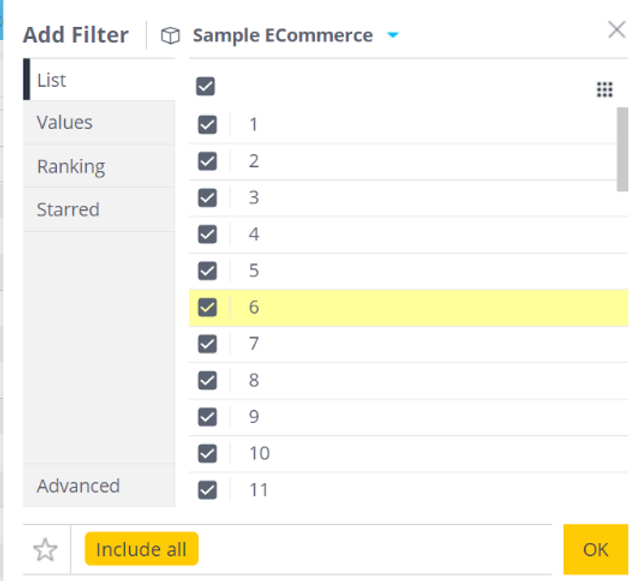
Levels of Filters in Sisense
Sisense offers three tiers of filtering granularity:
Dashboard Filters: Dashboard filters are applied at the dashboard level in Sisense, impacting all widgets within the dashboard. Users have the flexibility to modify these filters to suit their analytical needs. By adjusting these filters, viewers can refine the data displayed across multiple widgets, enabling comprehensive analysis tailored to specific criteria.
Widget Filters: Widget filters are applied within individual widgets, providing targeted data refinement within specific visualizations. When the same field is utilized in both dashboard and widget filters, only the widget filter takes precedence for that widget, overriding the dashboard filter. It's important to note that viewers do not have the ability to modify widget filters; only dashboard designers can make changes to these filters, ensuring consistency and control over data presentation within widgets.
Formula Filters: Formula filters are applied within analytical formulas and offer fine-grained control over data manipulation. When the same field is designated as a dashboard filter, widget filter, and formula filter, the formula filter takes precedence. This means that only the criteria specified in the formula filter will be applied, while the dashboard and widget filters will be disregarded. Similar to widget filters, formula filters can only be applied by dashboard designers, ensuring that complex data manipulations are executed with precision and accuracy.
Conclusion
Effective filtering is integral to extracting actionable insights from data within Sisense. By leveraging a combination of built-in filtering options and Paldi filter plugins, users can streamline analysis workflows, enhance usability, and unlock the full potential of their data. With the right filtering strategies in place, organizations can make informed decisions and drive business success.